
#ADVANCED OUTLOOK REPAIR 3.2 CRACK CRACK#
For example, in the work of Nahvi and Jabbari, the authors have combined experimental modal data and FEM to study the crack detection within a cantilever beam. Many research works have been done in the literature regarding this problem. One of the traditional methods that has been used for crack detection and propagation is the Finite Element Method (FEM). Consequently, the development of more robust and efficient techniques is crucial, aiming at saving time and cost for the maintenance of substantial concrete infrastructures, especially for those presumably exceeding their expected service life. Besides, the cost for the maintenance of substantial concrete infrastructure is currently expensive for instance, an average budget of five billion euros per year is used in Europe, as mentioned by the European HEALCON project, for the maintenance and repair activities. For existing infrastructures, especially concrete structures from the 1960s, this technique remains challenging. However, such monitoring technique is commonly integrated into modern construction facilities. Structural health monitoring mainly consists of using sensors to detect the changes in the stiffness of infrastructures as well as the initialization of corrosion. Īs the identification of cracks is crucial for the assessment of concrete damage, various techniques have been proposed for the maintenance of such infrastructures. Consequently, structural damage identification is shown to be inevitable to reduce the risks. Indeed, these cracks cause many problems for the reinforcement, such as corrosion and chemical attack. Notably, one of the significant impacts that severely affected the durability of concrete and reinforced one is the presence of cracks. However, these construction facilities are affected by concrete damage after years of service. Various infrastructures use concrete materials such as bridges, nuclear reactors, dams, and buildings.
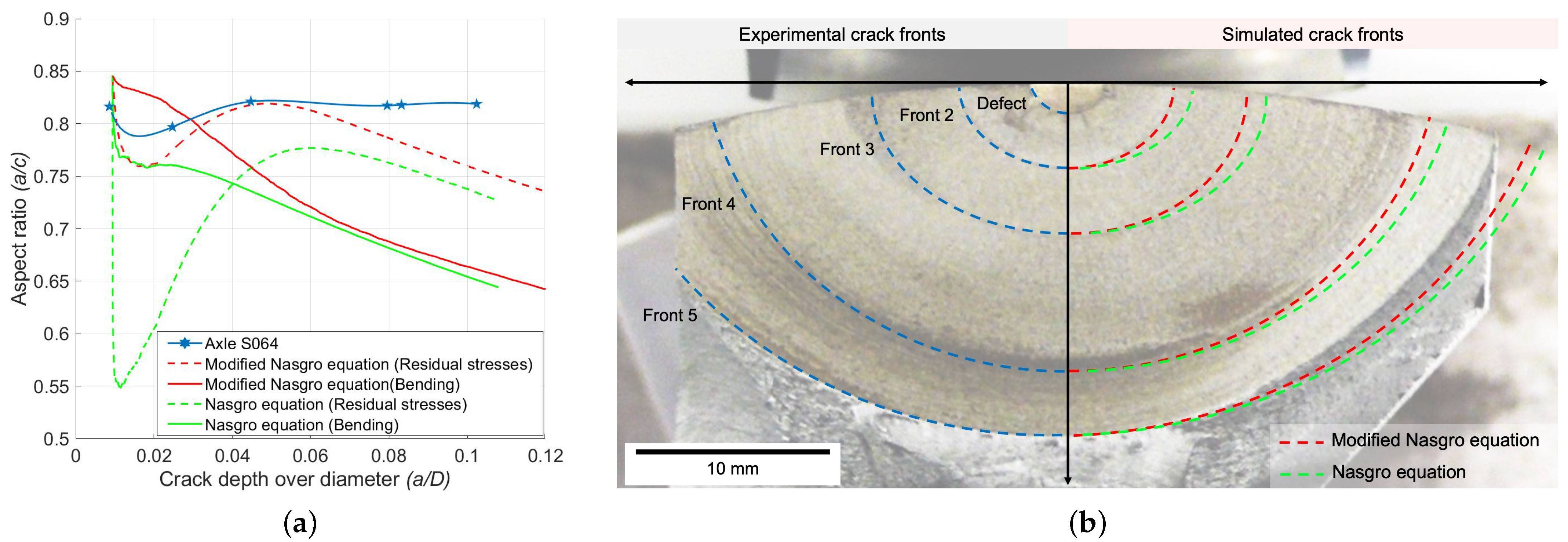
Finally, in further work, such model could be combined with Unmanned Aerial Vehicles (UAVs) to increase the productivity of concrete infrastructure inspection. The CNN model developed in this study was compared with other works in the literature, showing that the CNN model could improve the accuracy of image classification, in comparison with previously published results. It is also confirmed that the developed DL-based model was robust and efficient, as it can take into account different conditions on the concrete surfaces.
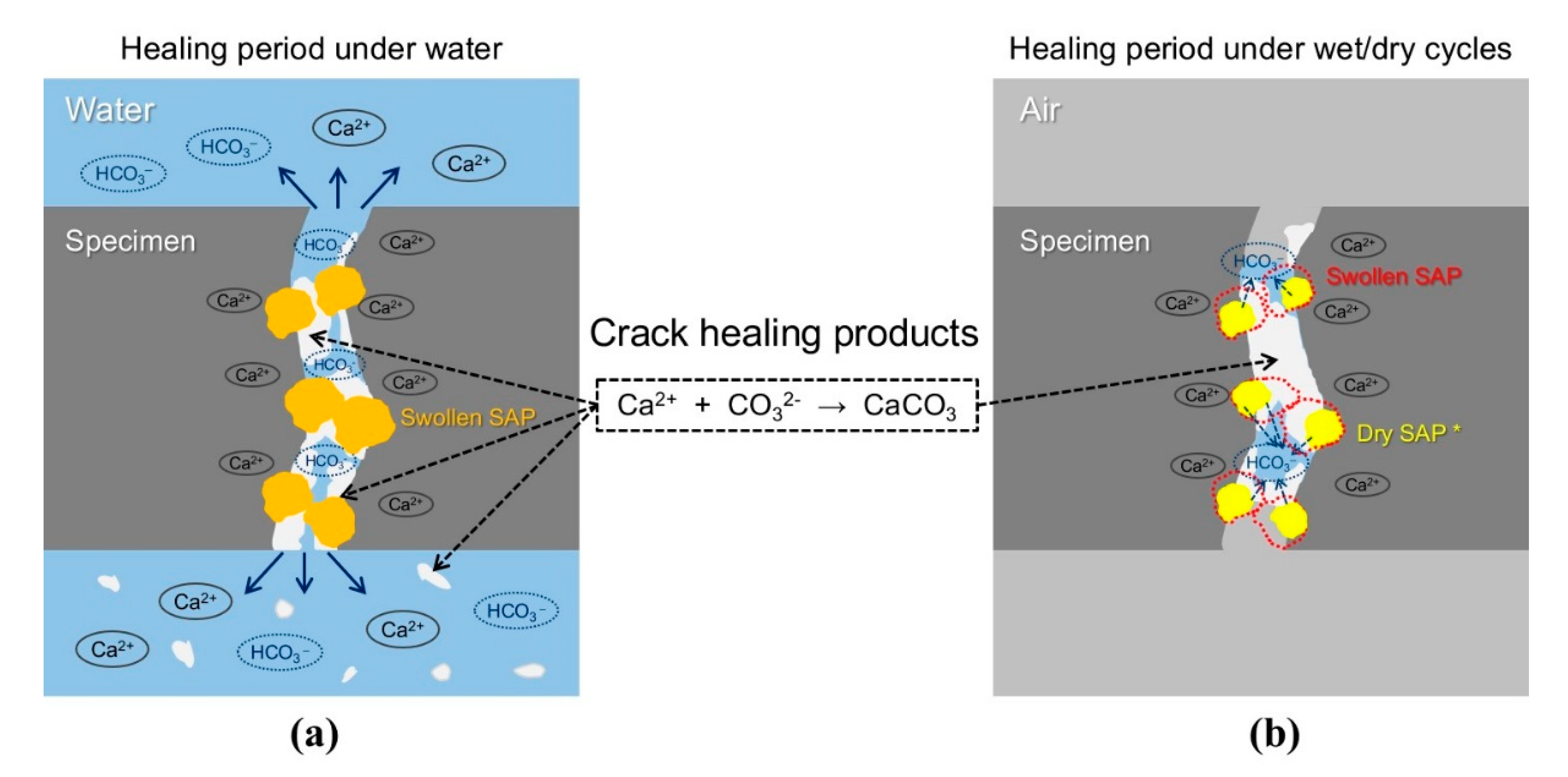
Thus, the developed CNN model may be considered valid because it performs the classification of cracks well using the testing data.
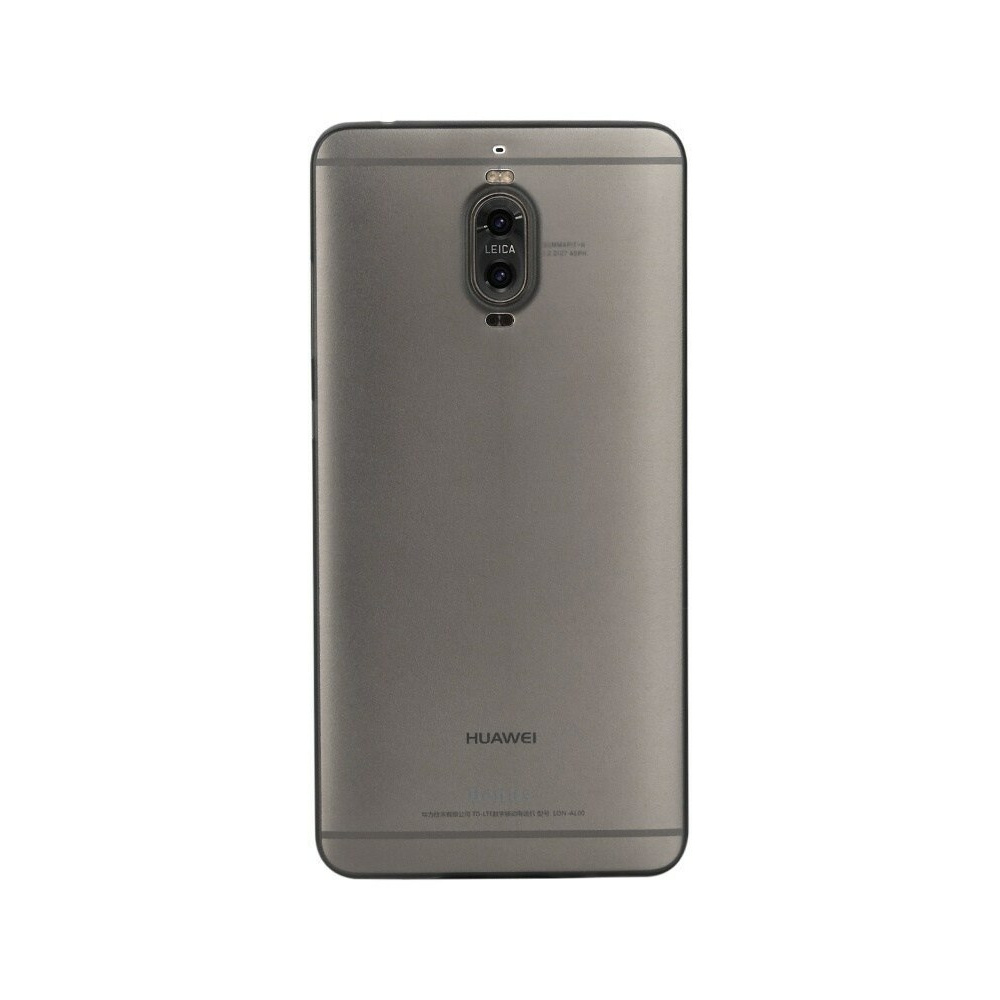
On the other hand, for the validating dataset, the precision, recall, specificity, F1-score, and accuracy are 96.5%, 98.8%, 96.6%, 97.7%, and 97.7%, respectively. Results showed that for the training dataset (50% of the database), the precision, recall, specificity, F1-score, and accuracy were 99.5%, 99.8%, 99.5%, 99.7%, and 99.7%, respectively. Various error measurement criteria such as accuracy, precision, recall, specificity, and F1-score were employed for accessing the quality of the developed model.
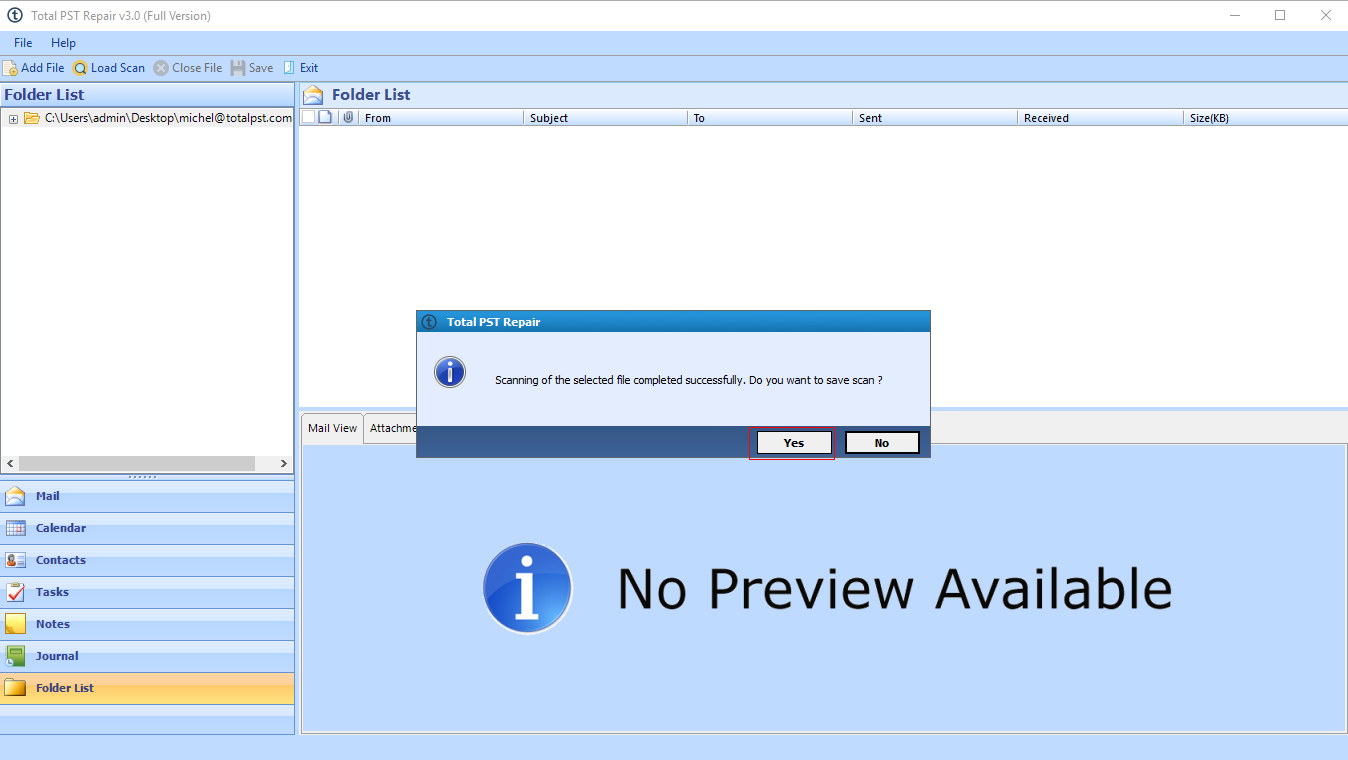
Several conditions on the concrete surfaces were taken into account such as illumination and surface finish (i.e., exposed, plastering, and paint). To train and validate the CNN model, a database containing 40,000 images of concrete surfaces (with and without cracks) was collected from the available literature. The developed model for the classification of images was based on a DL Convolutional Neural Network (CNN). This paper is devoted to the development of a deep learning- (DL-) based model to detect crack fractures on concrete surfaces.
